The AI-first unified platform for front-office teams
Consolidate listening and insights, social media management, campaign lifecycle management and customer service in one unified platform.
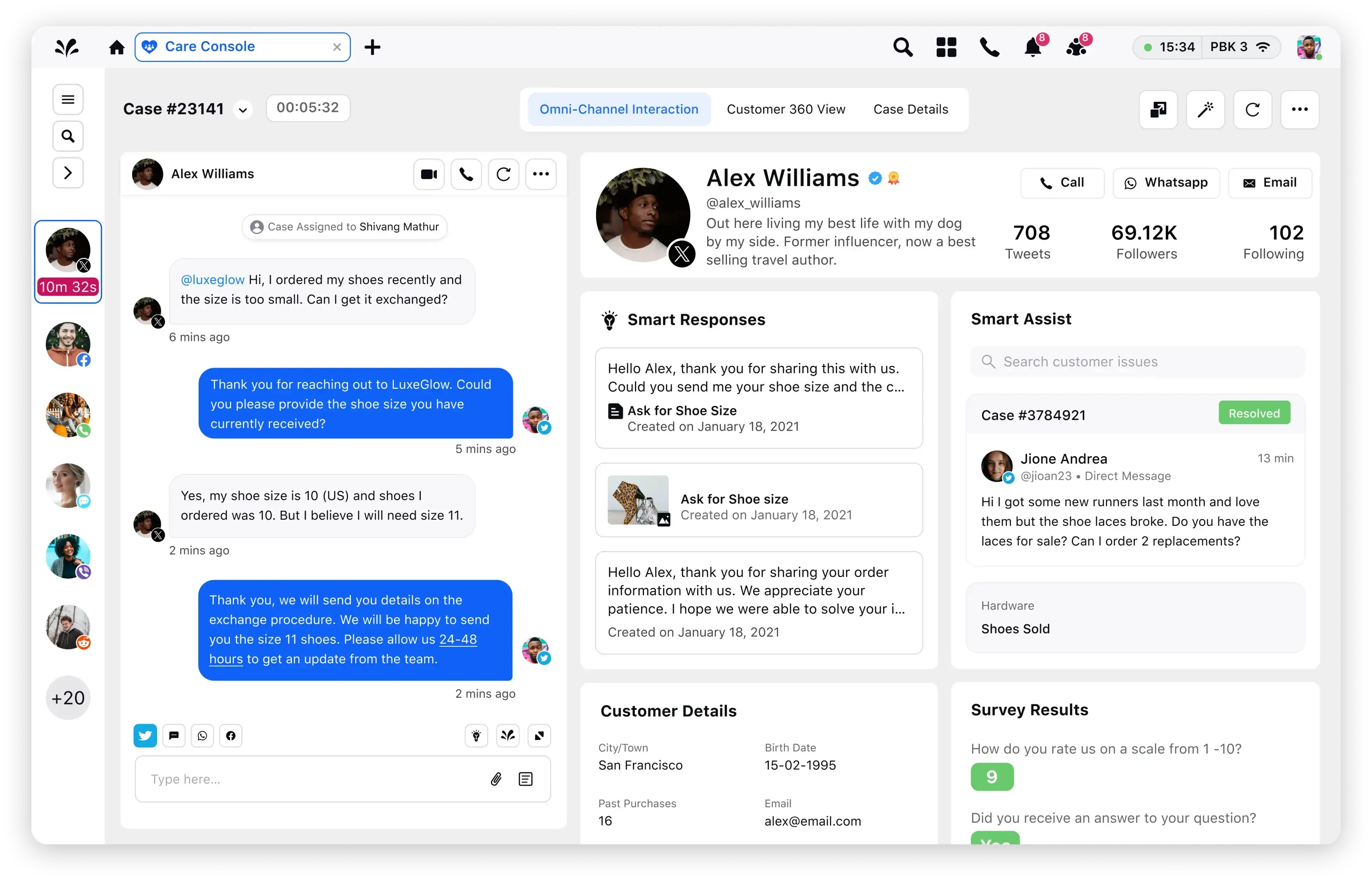
Democratize AI: 4 DIY use cases you can build using Sprinklr AI Studio
Modern brands are increasingly reaping the benefits of artificial intelligence (AI) technologies to drive their business. But, there are often challenges with out-of-the-box approaches. Namely, businesses in the SaaS industry frequently face prolonged timelines for creating custom AI solutions as well as a lack of ownership and governance over these processes.
This is why AI democratization is becoming a hot topic. There is both a desire and an increasingly urgent business need to allow average users to take advantage of AI solutions without having to connect with dedicated machine-learning teams. Sprinklr AI Studio technology is helping that vision become a reality. AI Studio lets users implement nearly limitless use cases, but, here are four examples of how customizable AI solutions can help you address the specific needs of your business.
Use case 1: brand disambiguation
Brand and/or product specific
Many companies own brands and products that incorporate common words, which creates challenges for social media listening. For example, consider the word "apple." Are you listening for mentions of the food or the brand? This use case is known as brand disambiguation. The listening queries these brands attempt to build often capture content that is irrelevant to their business. This, in turn, produces reports that do not accurately reflect the online brand perception, either from a conversation or performance perspective.
As a result, listening teams try to address this by investing more time maintaining their listening queries. Team members monitor captured mentions and enrich their inclusion and exclusion keyword lists daily. On top of that — and to ensure clean reporting that includes only actionable insights — teams must review these reports regularly, and exclude irrelevant mentions through manual tagging.
Ideally, brands need a solution that considerably limits the manual time needed to maintain these queries and reports, allowing their listening teams to focus their attention on more fruitful business activities. This solution should also produce clean reporting with relevant, actionable insights that teams can easily and quickly share.
Sprinklr AI Studio is able to address all of these challenges by allowing you to build custom AI models for every brand and product with common names. You can build a training dataset once, ensure that a model is performing ideally, and deploy the model to remove all irrelevant mentions with just one filter click. AI Studio eliminates the need for regular reporting check-ups and can expedite or automate report sharing without the need to check every message.
Use case 2: lead management
Vertical specific
Brand and product name listening volume can be enormous. To be more focused, brands try to slice and dice the data by using additional queries and keyword list themes that they apply as filters on top of their brand and product topics. By focusing on sub-areas that are linked to their social needs, team members can gain greater insights. But again, these filters will always end up catching irrelevant content.
Listening admins responsible for maintaining listening configurations must constantly update the theme queries. Over time, these themes become very complex and sometimes overly restrictive. Admins may try to revert to manual tagging. Generating leads from social conversations that happen outside owned accounts, known as lead management, is a common use case that requires these intensive configurations. But building and maintaining them is challenging, in part, because of the inability of boolean-based configurations to capture the contextual differences.
Sprinklr AI Studio can help bypass all of these issues. Clients can build their own verticalized AI models to capture conversations that would trigger action. Users can take it a step further and create their own custom lead management AI models that offer detailed lead subcategories.
For example, telecommunication companies interested in capturing general leads can subcategorize such conversations into upsell opportunities, including upgrading services and offering renewals. Additionally, they can act on churn threats, deactivation requests, and requests about downgrading services. They can also identify opportunities to win business from competitors by listening and categorizing their competitors’ public customer conversations. This allows the brand to effectively and quickly route leads to the appropriate teams to boost its sales pipeline and ensure leads don’t get lost. Here are just a few examples of lead management subcategories:
- Upsell opportunity
- Upgrade
- Competitive switch
- Churn threat
- Renewal
- Deactivation
- Downgrade
Use case 3: spam management
Vertical: vertical-specific
Brand: brand-specific
Apart from the usual ads and irrelevant commercial content, spam can include additional custom client-specific categorizations depending on their reporting and engagement guidelines. Sometimes, spam is identified differently from one company team to another, making out-of-the-box spam classification models ineffective. With AI Studio, companies can create their own AI models to address spam filtering. By giving end-users the power to create their own models on the spot, AI Studio helps shorten these extended implementation timelines. This also ensures that your brand is in control of what gets labeled as spam and what doesn’t. A spam classification example within the banking sector may be to “include all stock-related messages.” Some banks would like to keep these messages in their listening purview, while some prefer to eliminate them.
For a business like retail electronics, users may want to eliminate content posted by resellers or anyone not directly affiliated with the original company. AI Studio is able to detect patterns and provide only the information the user needs.
Use case 4: emotions framework
Vertical: vertical agnostic
Many brands are starting to look beyond sentiment analysis. Whereas sentiment analysis can reveal general negative, positive, and neutral brand perception, companies want to dig deeper into the emotional perception of their product. They want to know when a customer is sad, angry, fearful, or joyful about an experience. This gives companies more detailed insights into what’s working and what’s not in their customer experience.
AI Studio can categorize messages into a variety of brand-defined classifications, moving beyond general sentiment. Key benefits include:
- A unified approach to emotions and analysis across teams, channels, and practices
- Deep understanding of consumer perceptions around a brand or industry
- Consistent monitoring and maintenance of the models without needing to employ a third-party data science team
Bonus use case: own your languages
Sprinklr AI Studio now supports 101 languages, allowing local teams within global companies to participate and expand their global listening capabilities.
Developing and maintaining integrated social listening queries and manually tracking relevant social mentions can be both cost-prohibitive and time-consuming. Learn how Sprinklr's custom AI models can quickly and easily help you create unique and actionable insights for your brand.