The AI-first unified platform for front-office teams
Consolidate listening and insights, social media management, campaign lifecycle management and customer service in one unified platform.
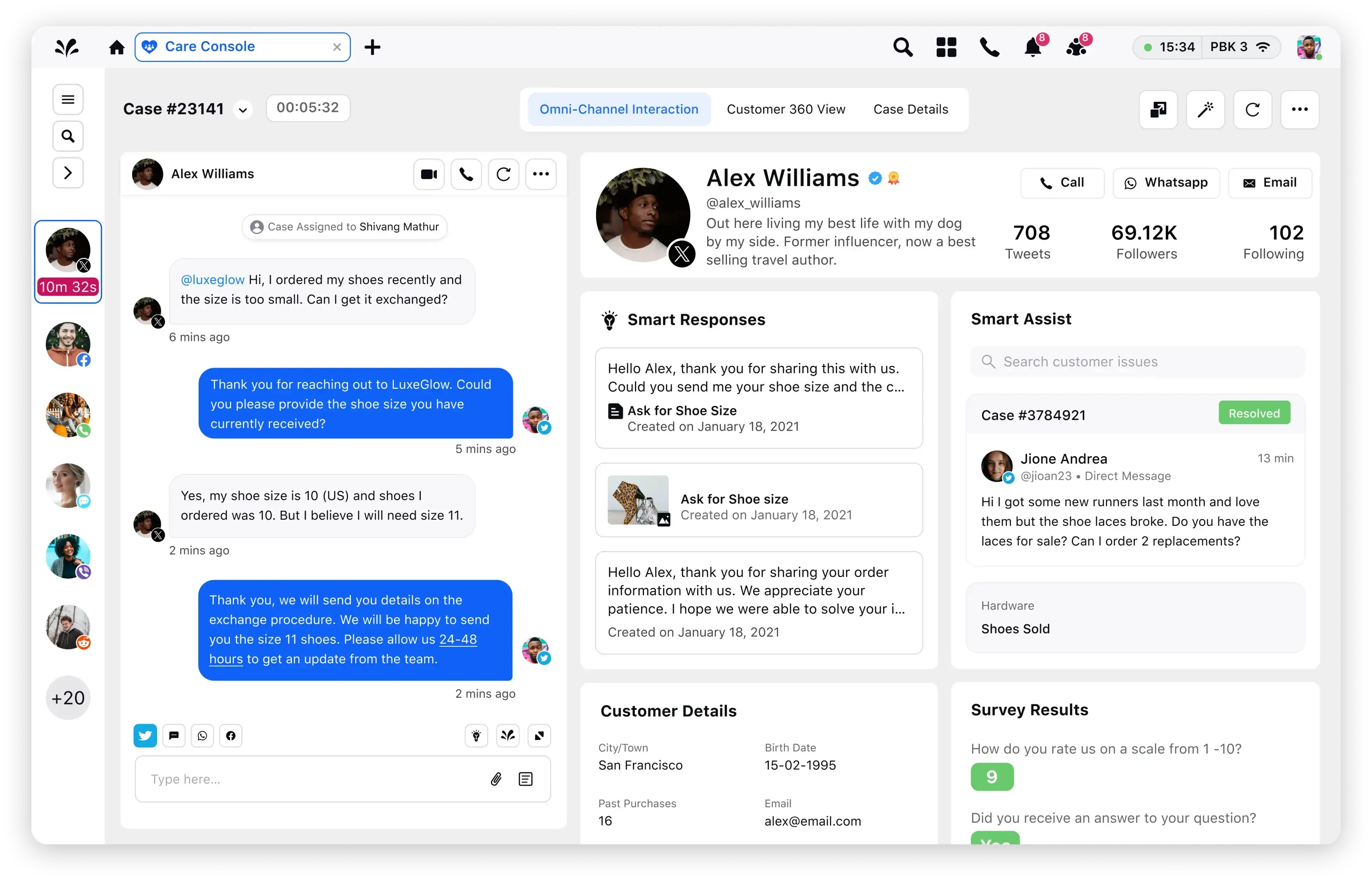
How Sprinklr Leverages Advanced RAG to Unlock Generative AI for Enterprise Use Cases
In today’s rapidly evolving AI landscape, the quest for delivering precise, up-to-date, and contextually relevant responses has led us to Retrieval Augmented Generation (RAG) frameworks. RAG enhances large language models (LLMs) with the ability to fetch factual knowledge from a wide array of external data sources. This approach not only increases response coherence but also significantly improves overall response quality by surfacing relevant brand-specific data, including documents and images.
Bridging the knowledge gap with RAG
Traditional LLMs, while adept at generating coherent narratives, often falter when tasked with delivering deep, factual insights on specific (or specialized) subjects. This limitation is further exacerbated when the model is dated or lacking in the ability to source relevant information.
The traditional challenges posed by out-of-date, sourceless LLMs - including inaccurate responses, contextual irrelevance and potential misinformation - are effectively mitigated by RAG's dynamic data integration and sourcing capabilities. This not only enhances trust and reliability in AI-powered applications but also ensures cost efficiency by circumventing the need for frequent, expensive retraining of foundational models.
RAG is able to resolve all of these challenges and more:
- Cost: RAG is a cost-effective alternative to the prohibitively expensive process of retraining foundational models, by virtue of its seamless incorporation of domain-specific information.
- Relevance: With RAG, LLMs can tap into the most current data from dynamic sources such as social media and news platforms, ensuring response relevance.
- Trust: RAG is able to cite its sources, improving explainability and driving greater confidence regarding the accuracy and reliability of the information provided.
- Customizability and control: RAG allows for meticulous curation of information sources and management of sensitive content, aligning the LLM’s output with organizational standards and security protocols.
Sprinklr RAG: How is it different?
What truly sets Sprinklr's RAG solution apart is its comprehensive, enterprise-focused approach. Sprinklr RAG is designed to seamlessly integrate with an organization's existing knowledge management systems, providing a unified platform that enriches AI interactions across various applications. The inclusion of an orchestrator module transforms AI from a mere information provider to an actionable insight generator - capable of executing tasks and facilitating a more interactive user experience.
Security, ease of implementation and customization are core to Sprinklr's RAG framework. The platform's architecture ensures compliance with the most stringent security protocols while the user-friendly design enables seamless integration and scalability. Moreover, Sprinklr RAG offers flexibility in LLM and search engine customization, accommodating the unique needs of each enterprise.
Sprinklr’s RAG architecture
Sprinklr’s RAG architecture ensures a robust and effective solution. The AI Search feature, powered by Sprinklr AI, ensures accurate and fast retrieval of information from a multitude of sources, employing a re-ranking strategy for relevancy and incorporating both dense and sparse embeddings for semantic searches. The Knowledge Base component not only supports a wide range of data formats but also provides insights to mitigate misinformation, with customizable prioritization settings for different knowledge bases.
The platform's customizable guardrails offer unparalleled control over content generation, with global settings for user input moderation and topic-specific controls for source and content management. Moreover, the tools and APIs supported by Sprinklr RAG extend its functionality, enabling integration with external services for enhanced user routing, news retrieval and product recommendations.
In terms of LLM provider flexibility, Sprinklr stands out by supporting a variety of models, including in-house, open-source and enterprise options. This agnosticism, coupled with the BYOK (bring your own key) feature, allows organizations to integrate their proprietary models, ensuring that AI solutions are perfectly tailored to their specific requirements.
Realizing the full potential of Generative AI with Sprinklr RAG
With Sprinklr, enterprises stay ahead of the AI curve while delivering exceptional experiences to their users and customers.
In a world where the right information at the right time can make all the difference, Sprinklr RAG truly empowers organizations to harness the power of Generative AI and redefine the boundaries of what is possible.
Future trends
- Sprinklr is poised to leverage advancements in AI to further refine its RAG solutions, focusing on improved accuracy, deeper integration capabilities, and enhanced user interaction.
- Sprinklr recognizes the dynamic nature of this field and is committed to continuous innovation. The platform regularly expands its offerings to enhance user experiences across various scenarios, ensuring it remains at the forefront of AI.
Call to Action
- Organizations looking to stay ahead in the rapidly advancing realm of AI and knowledge management are invited to explore Sprinklr's RAG offerings.
- By choosing Sprinklr, enterprises can unlock the full potential of generative AI, ensuring they not only keep pace with technological advancements but also deliver exceptional experiences to their users and customers. Sprinklr AI+: The power of Generative AI, within Sprinklrread more
