All great brands know that a flawless customer experience is key to competitive advantage. According to Gartner, businesses that prioritize value-driven customer service interactions are more likely to retain customers (82%), get repurchases (86%), and have positive word-of-mouth (97%).
But not all customers and their requests are created equally. When a customer approaches a company, the representatives who attend to them act on the company's behalf. But how does one ensure that the right representative is assigned to handle a customer's needs? And most importantly, how will customers perceive the quality of service they receive?
Contact centers that handle complex queries and rely on large teams often use standard case assignment mechanisms that factor in constraints like agent availability, capacity and skills, among others. However, such approaches may not always be optimal.
Challenges with traditional case assignment methods
First, skills, such as proficiency in solving a specific issue or question, are manually assigned, hence subject to bias that the supervisor might introduce into the process. They also require frequent manual updates to be relevant.
Second, contact centers might overlook perceived aspects of agents' performance when assigning them to customer requests. For instance, during your last interaction with a brand you may have noticed that an agent was either patient or impatient. You might also have observed that they communicated poorly.
Did you have a chance to let the brand know? Perhaps yes, in an aggregate form. But providing explicit feedback about single facets of customer service is often infeasible, as it requires extra effort. For these reasons, brands don’t usually have a feedback system that takes into account all of these aspects for all of their interactions; and - even so - the feedback is not looped back to influence the assignment strategy in real time.
Third, contact centers fail to consider some pertinent details about agents and customers, including demographic, behavioral, and psychographic information.
For instance, a recent analysis of customer electronics cases revealed that elderly customers tend to value specific agent characteristics, such as patience. Although legal and data usage constraints may exist, there is a need to do more in understanding customers and their preferences.
How AI-powered Smart Pairing can help
Intelligent routing strategies are a breath of fresh air in an otherwise rigid process. They work on both text and voice and rely on assignment algorithms that help brands assign the best agent to a case, with an overall better experience than the one obtained with manual skills updating:
Both new and returning external customers benefit from increased personalization, less handovers, faster turnaround time and an overall smoother experience
Internal customers (agents) are empowered, as unique aspects of their expertise and behavior are leveraged where and when it matters the most. They also benefit from less handovers and escalations
Internal customers (Care supervisors) save time by not having to update agents’ skills manually, and can rely on up-to-date and unbiased information at all times
Smart Pairing, Sprinklr AI-based automatic assignment algorithm, can help brands improve the customer experience by assigning the most suitable agent to handle a case. The solution can be tailored to specific needs and available data. Some of the information that can be leveraged includes:
Intent proficiency score over time: If a survey is sent to customers at the end of a case to rate an agent's performance, Smart Pairing takes this feedback into account, associates it with the type of query the agent resolved, and updates their overall score.
This information can be used to route future queries to the most suitable agent. For example, if Agent A excels in resolving "refund” queries' and Agent B is better at handling "opening time" queries, Smart Pairing will prioritize routing these queries to the respective agents, even if both are qualified in both topics according to manual assignment.Perceived service quality score: For example, while conversing with Agent A, a customer may comment, "Everything is super clear," but also express dissatisfaction by saying, "Why can't you be more flexible?" or leave feedback in a survey stating, "The agent spoke good English but didn't provide any customized resolution."
In this case, Smart Pairing automatically identifies Agent A as proficient in communication but less proficient in flexibility, which influences future pairing decisions.Segmentation data: Smart Pairing can also take advantage of traditional demographic, behavioral and psychographic data for both agents and customers, as well as emotional cues from speech, whenever applicable.
Contextual information like date and time.
How Sprinklr’s Smart Pairing skyrockets your customer experience
A well-executed call routing strategy can bring substantial benefits to both customers and agents, including improved experiences, higher retention rates, and increased revenue. To achieve these results, businesses must rely on advanced routing engines, which offer skills-based, data-driven, and omnichannel routing capabilities. With Smart Pairing, organizations can further enhance their call routing capabilities with the power of AI.
The algorithm blends in seamlessly with best-practices Sprinklr Services set-ups, and our teams are ready to support you in every step of the process, from sourcing data to fine-tuning of the algorithm to maximize accuracy for specific contexts. The algorithm automatically re-calibrates for each new input and updates itself regularly to ensure that the strategy doesn't overlook any new insights.
Smart Pairing consists of two steps: Scoring and Pairing.
STEP 1 - Scoring
For each closed case and involved agent, intent resolution proficiency is automatically calculated on the basis of the detected intent and the most suitable proficiency metric available for the customer’s data.
For example, the system may use customer survey scores, Sprinklr predictive CSAT, or customer sentiment intensity to determine the proficiency of an agent in handling a particular type of issue. The specific metric(s) used and the strategy for combining them is determined on a case-by-case basis after conducting a preliminary assessment.
For example, if for a certain brand, a high correlation between two proficiency metrics is observed, such as predictive CSAT and numerical survey scores assigned by customers, either metrics can be used as a good predictor of agent performance. In situations where numerical survey scores are missing for most cases, predictive CSAT can be used to supplement them.
Agents can also be scored based on perceived service quality factors such as Access, Accountability, Accuracy, Communication, Courtesy, Efficacy/Effectiveness, Efficiency, Flexibility, Knowledge, Patience, Promptness, Interest, General Positive Experience, and General Negative Experience. These scores are based on mentions found in case messages and reviews, if available. For example, an agent's score for "patience" may increase if a customer mentions that they were patient in a case message or review.
Other scoring strategies based on segmentation data and contextual information (if available) can also be considered.
Scorecards are periodically updated.
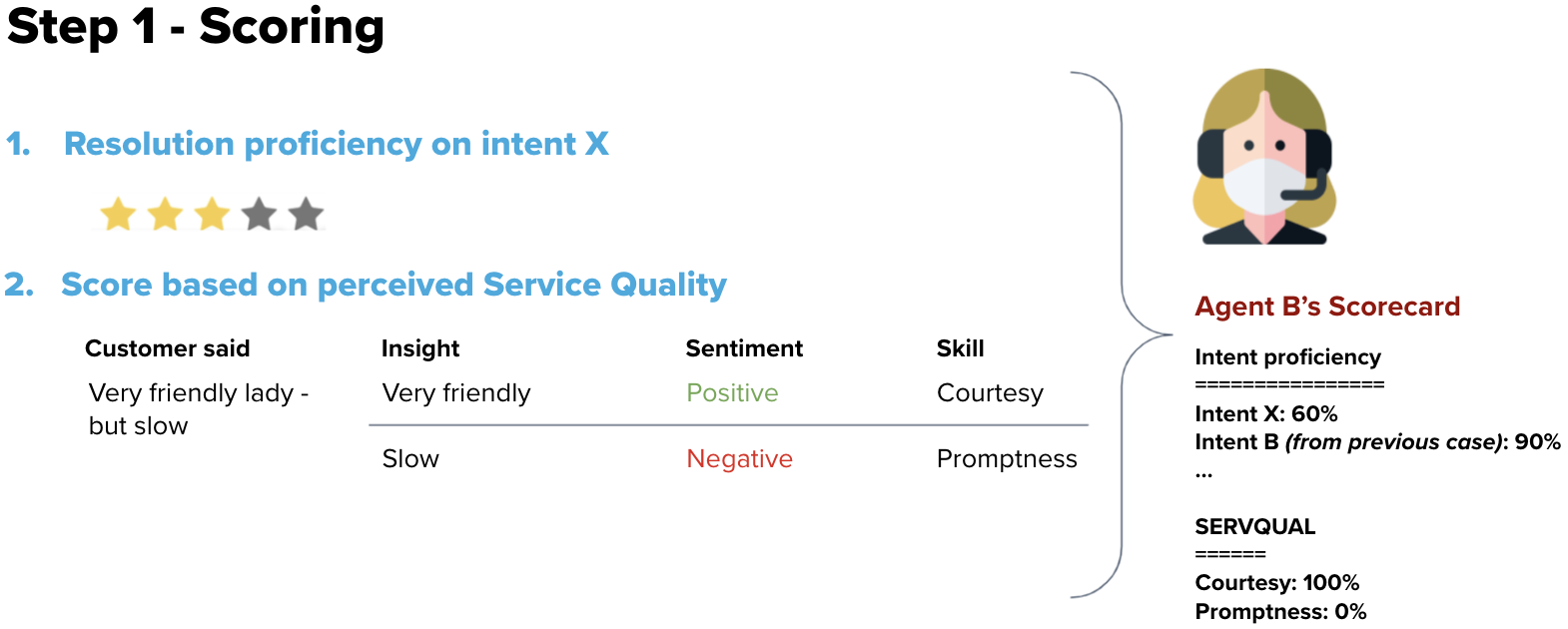
STEP 2 - Pairing
In the Pairing step, Sprinklr Smart Pairing assigns the most proficient agent to a new case based on their historical and ongoing intent proficiency and/or perceived service quality scores. Other pairing strategies based on segmentation data and contextual information (if available) can also be considered.
Instead of standard pairing strategies, usually relying on manually assigned skills and FIFO strategies, the case will go to the agent who is best matched, regardless of whether another compatible (but not optimal) agent was available first.
Anti-bias measures are implemented to ensure that all agents are treated fairly, meaning that even agents who are less proficient in a particular intent will still receive cases related to that intent occasionally, giving them an opportunity to improve their score.
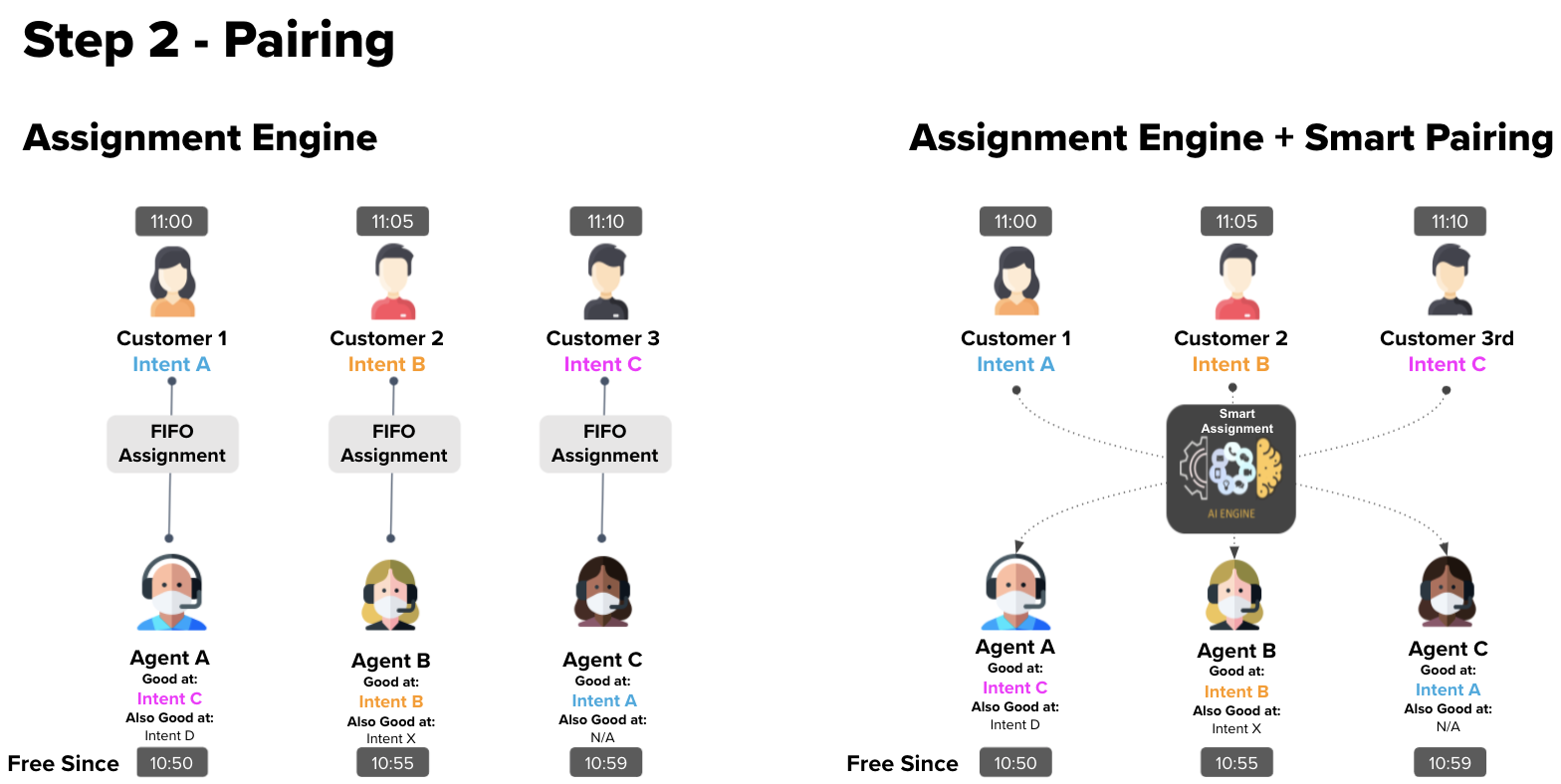
Additionally, Smart Pairing also works well in two typical care scenarios that require further attention:
Overloaded work queues: Smart Pairing can effectively handle overloaded work queues, which is a common care scenario (cases accumulate into a large backlog). Traditionally, the approach is to assign the first available agent to the oldest waiting case. Smart Pairing, on the other hand, considers the subset of cases in which the available agent would perform best based on the chosen score, and selects the oldest case from that set. However, to avoid unacceptable delays, a maximum wait time can be set, after which a case would be given the highest priority, overriding the Smart Pairing logic.
Multiple agents within the same case: Smart Pairing employs different strategies based on the situation.
Equal assignment: all involved agents are scored equally
Proportional assignment: the score is assigned proportionally, based on the level of involvement of each agent
Threshold-based assignment: a certain minimal threshold is set (e.g., the agent must have contributed with min. 3 messages in order to be scored). Only agents with a minimal threshold receive the score, equally or proportionally
The ideal strategy can be selected automatically, based on the outlook of the training data, or manually.