The AI-first unified platform for front-office teams
Consolidate listening and insights, social media management, campaign lifecycle management and customer service in one unified platform.
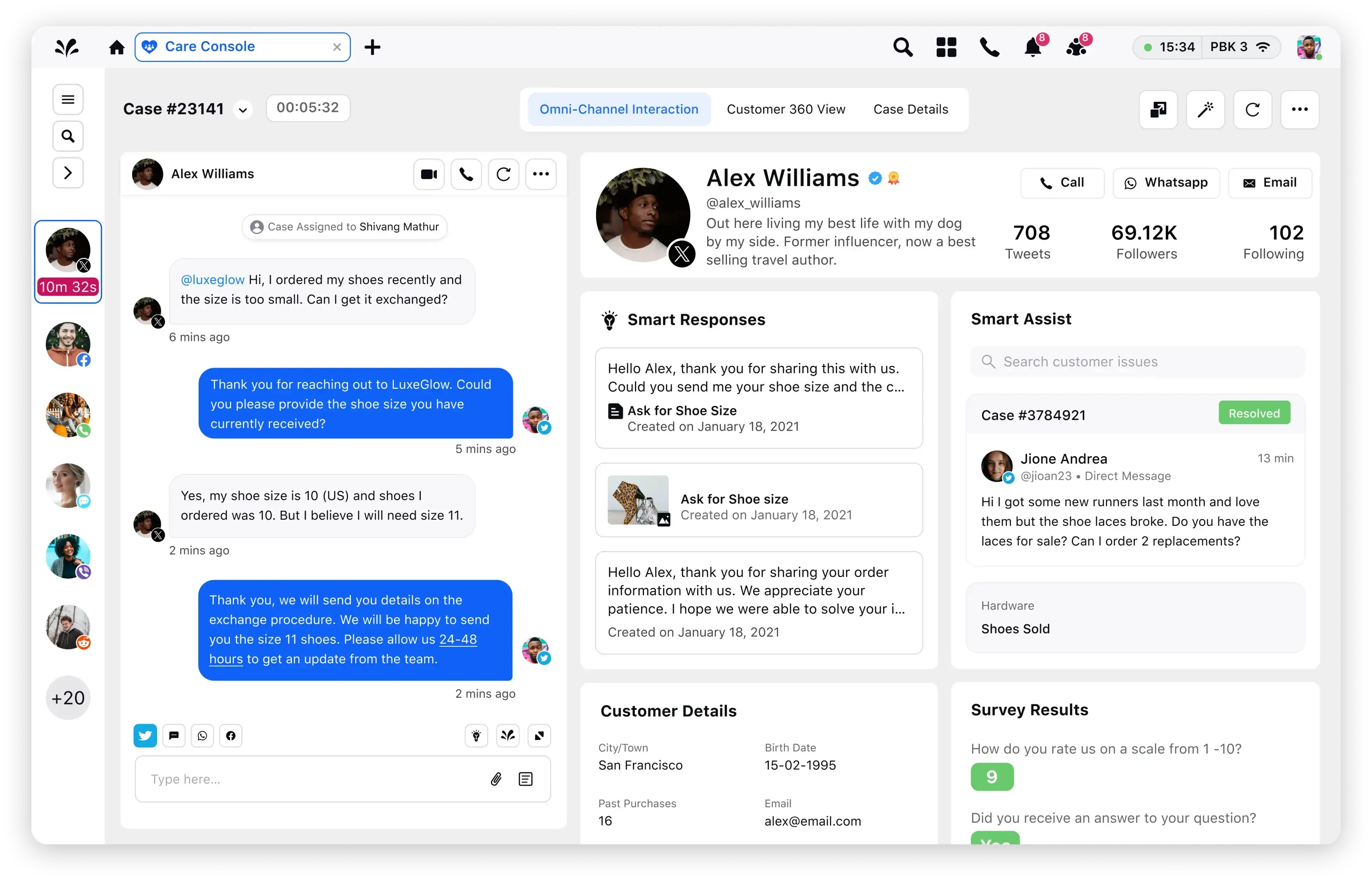
We were promised effective Self-Service. What went wrong and what needs to change?
Martin Hill-Wilson, a prominent thought leader in the customer service and CX space, candidly shares his thoughts on designing a self-service system that helps customer reach their outcomes faster.
Optimising a contact strategy is one of the most critical strategic leadership challenges.
“The right mix of live assistance, self-service and proactive messaging determines cost-to-serve, resolution speed, and resolution quality, to name a few of the more obvious benefits.”
Central to this rebalancing is allowing customers to self-serve. It’s something they want. Provided, of course, it works.
The reality for many customers, though, is that self-service fails to deliver the outcome they expect and still requires the intervention of a person. Why does this remain such a commonly reported customer experience? Against this general perception are award-winning brands that are getting it right and managing to scale self-service to meet more than 60% of their customer demand.
I’m going to explain why technology is not the issue. Instead, it’s an organisational and cultural challenge.
Living in a World of Self-Service
There is nothing new about self-service. Taking humans out of the loop has been ongoing at different speeds and use cases in most countries for decades. Some versions are so well established that users no longer recognise the novelty. Or even know it used to be different. In the UK, examples include refueling at a petrol station or accessing banking services via an ATM.
These types of self-service enjoy users’ support and widespread adoption because they get what they want quickly with little effort. Moreover, knowledge of how to operate them is widespread and easy to discover.
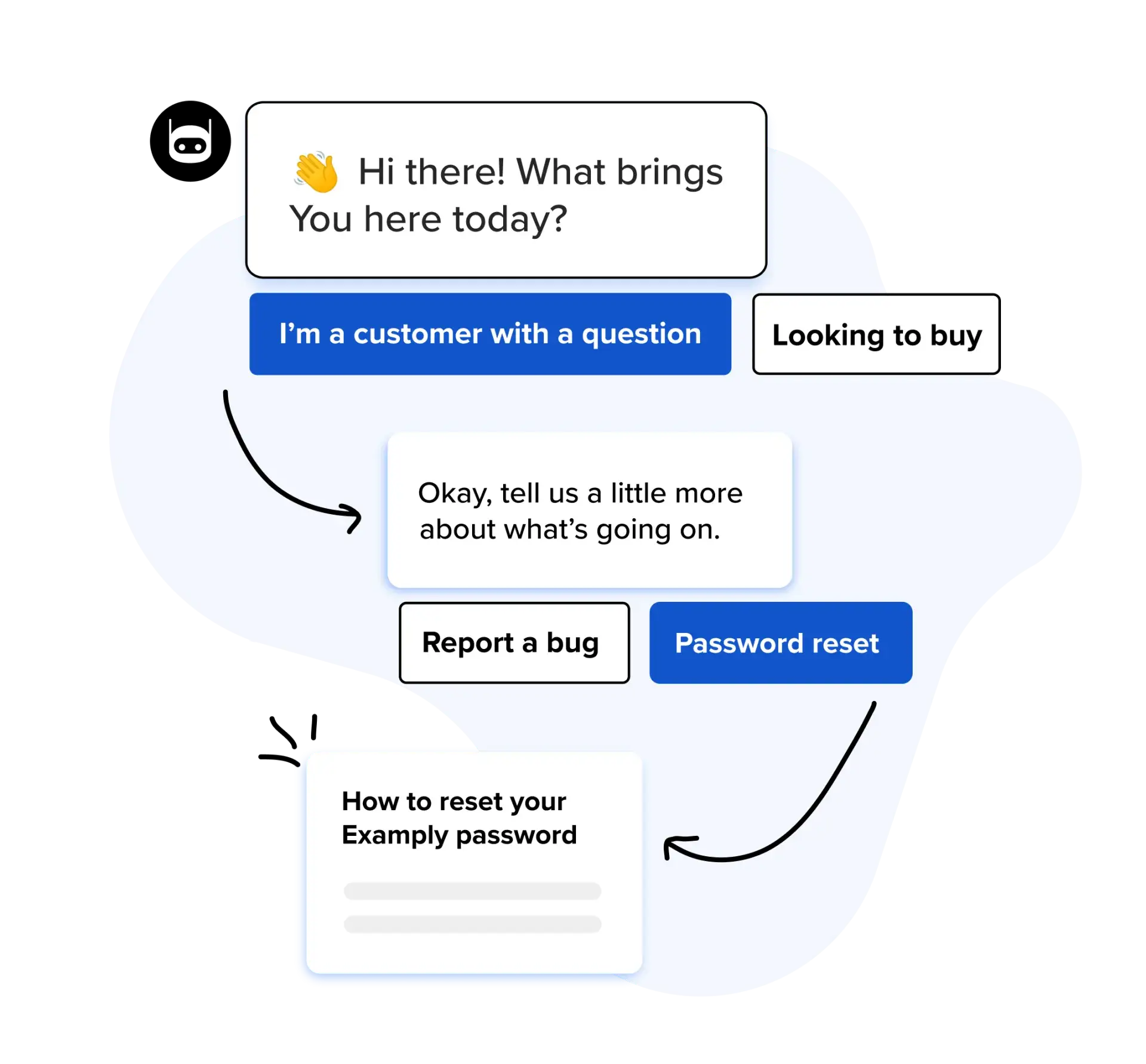
However, there is always a new frontier in the quest to remove the human touch. Five years ago, I was approached by a CEO running a customer service BPO who wanted me to advise them on how to swap all their live assistance for a Conversational AI-powered alternative. I declined. I recently heard a similar request. This time – it is about Generative AI.
I can’t fault their disruptive instinct – just the lack of insight into what it takes to outsource an outcome back to customers successfully.
It is easy to forget that human-to-human interaction is a process of constant adjustment, iteration and sense-checking since what comes out of our mouths seldom matches what sits in our minds. And we infrequently finish one idea before another one pops out.
Most people learn to recognise these logic gaps and inconsistencies with a life-long, honed ability to infer meaning. And instinctively factor in any relevant context about the situation, the topic or the person. This sense-checking is augmented with how our bodies react and the emotive clues generated to help us better sense underlying meaning and significance. In short, a lot is going on while understanding one another.
The degree to which this array of human sensors is activated in customer engagement depends on the topic. Discovering the location of the nearest store and its closing time is a simple task with few steps. Even so, that simplicity fades if the nearest one is miles away or if it closes before you are likely to reach it.
What happens when we ramp up the complexity, urgency, and emotive nature of a customer need? For instance, needing to re-negotiate monthly payments given a cost-of-living crisis. Most contact centres make this a prime use case for specially trained colleagues. And yet, even in this situation, some customers will opt for the anonymity of a chatbot when discussing personal matters with strong emotive undertones. Many of the customers' issues and frustrations with self-service lie between these extremes.
Here are a couple of initial guidelines based on what we discussed.
- Ignore the complex stuff until you have put significant effort into simplifying the process of discovery and resolution. As previously explained, complicated discussions that can flow any which way are not easy to design for, even when Natural Language Understanding is on tap.
- Never assume that ‘simple’ cannot convert into ‘complex.’ Or that ‘rational’ cannot transform into ‘emotive.’ We can never fully anticipate what will happen to a particular customer in a specific situation. So, make the pathway to human support visible, easy to achieve, and avoid customer frustration of needing to repeat themselves.
Organisations predicting a self-service investment solely on the ROI of reduced ‘cost-to-serve’ find this logic hard to stomach.
“It’s why the language of ‘deflecting contacts’ still exists. The mindset comes from a brand-centric priority to reduce costs rather than a customer-centric view of delivering greater benefits.”
Incidentally, this is one of the prime reasons IVR suffers such a poor reputation – from a widespread perception of adding obstacles to customer outcomes rather than facilitating them.
Design for Virtual and Human assistance teamwork
Self-service rises and falls based on the use case and setting the right balance between automation and human intervention. For instance, a few people will have been in a human-operated lift these days. They once existed in top-end department stores. At least in the movies! Now, we use them without question in self-service mode. But notice they still offer access to human assistance in anticipation of something going wrong.
And there are reasons other than the unexpected as to why we still need a human in the loop.
For instance, think about supermarket self-checkout tills. If you are familiar with paying via a cashier, you might think automating can’t be too hard. Empowering consumers can only multiply the number of checkout points and reduce queuing. Indeed, that’s a win-win.
Turns out things are not always that simple. Users can double charge themselves and need an item removed. They might need help pricing unwrapped fresh produce without a barcode. Or end up slowing down the queue looking up an item. Is rhubarb listed as a fruit or vegetable?
With the benefit of such hindsight, most UK supermarkets still offer their customers a choice of waiting in a longer queue for a cashier to do the processing and sometimes lend a hand with the packing. Or join a faster-moving queue that allows customers to do the scanning under the expert supervision of staff who constantly keep the queue moving by removing the glitches caused by people, processes, and products. It’s pragmatic and seems to work for now.
Of course, more ambitious disrupters see things differently. Amazon Go is built on the belief that this layer of supervisory support can be removed entirely with sufficient high-tech wizardry using computer vision, deep learning algorithms, and sensor fusion.
They have meticulously modeled real-world human behaviour to ensure customers can be in and out of their store within 90 seconds and that Amazon gets paid in full. They are experts at mitigating user effort, so in time, their version of cashier-less retail becomes the default.
But here’s the point. Customers both welcome and fear these changes. We are using technology in increasingly ambitious ways to reshape o
ur daily lives. Seeing a food-home-delivery service enabled by an army of robot pickers is a feat of algorithmic genius and clever technology fusion. We are on track as a society for large-scale automation. Generative AI now extends this to white-collar work.
In previous large-scale automation cycles, we see certain job types become redundant and others invented to help manage the technology. At the same time, entirely new opportunities are birthed through the creative disruption unleashed.
In an age in which CX still drives loyalty and commercial success, making the right judgement about where human assistance sits and how much is needed and remains visible to the customer is critical in this broader context of our reaction to automation.
Even though the temptation to rely exclusively on automation to boost P&Ls in harsh trading conditions is understandable, it ignores the complexities of life. Experts still supervise the robot pickers to sort out edge cases and optimise their workflows. While the advice most seem to be settling on at this point for the ‘safe’ use of Generative AI within organisations is to have humans in the loop as quality assurance checkers.
Technology innovators have an unfortunate habit of being far too euphoric about benefits and time to value forecasts. Being excited in front of an audience who have little context with which to judge this ‘amazing new thing’ is a recipe for broken promises, leading to the so-called ‘trough of disillusionment’ with the expense and lost time of having then to win back those with memories of poor self-service experiences.
Review any relevant conference, webinar, or roundtable agendas over the last decade, and the sources of disappointment are clear to see. Self-service technologies were evangelised as the answer to efficient and personalised customer service.
Instead, the quality of service often declined as human assistance was removed too fast. Or digital-first initiatives were naively pasted onto existing workflow, and customers had to complete in traditional live assistance channels.
And that’s before buggy interfaces, lack of intuitiveness, and an over-reliance on customer self-sufficiency combined increased frustration and decreased satisfaction. We discovered that a poor reputation amplified across social and traditional media can turn off a whole generation.
So, how do we do a better job moving forward?
One of the ways is to set more realistic claims and acknowledge from the outset that self-service and human support always go together, as I’ve been illustrating.
Let customers know that virtual assistance has a human counterpart. It’s a team effort. Stating this upfront does not threaten ROI. In fact, it accelerates it for the following reasons.
If you have correctly identified a viable self-service use case, then designed and optimised it to work so that the journey is now clearly superior to waiting in a queue for live assistance, you will only need to respond to the occasional customer request for human support.
You are satisfying a widely established habit of digital self-sufficiency. In other words, the need for human assistance is removed even though you have offered the psychological comfort of offering human support. People gravitate towards any path of least resistance. All you need to do is make the self-service option faster and easier.
Alternatively, if you find yourself stuck with low self-service adoption and many requests for human assistance you have actionable feedback. Either you have chosen the wrong use case or the solution you have designed is falling short of needs and expectations and customers quickly give up.
This gives us another rule of thumb in how to make self-service a preferred customer option.
Self-service channels need constant attention to remain fit for purpose, for instance, ‘stuck in a loop’ IVR experiences, bots that fail to recognise a new intent, and missing workflow that causes the transfer to live assistance.
‘Drink your own champagne’ should be a daily activity for every self-service owner. Don’t rely on reporting to tell you what’s going on.”
Sample the journey and all its variations just as a customer would. Then, you will understand by duplicating their experience why they still want to engage a human to help them.
In summary, promote virtual and human assistant teamwork and remove any perception your motivation has been to make a customers’ life any harder. Develop feedback loops that are highly responsive and result in shortfalls being fixed as fast as possible since getting users to resample something that has failed them is next to impossible. To guarantee that level of responsiveness, the best model I’ve come across is cross-functional teams working within an agile framework.
Building Winning Teams and Ways of Working
If IVR taught us anything, it is unrealistic to expect experts in the technology to be experts in how customers use it. A more diverse set of skills are required. This applies to any self-service mix you develop utilizing the building blocks of natural language, intelligent search, next-best-action algorithms, FAQs, voice/text bots, avatars, AR/VR, kiosks, portals, apps etc.
Here are the most important skills I’ve noticed in the award-winning teams I’ve judged. These are the skills they typically base their cross-functional team on. All use an agile methodology as an organising principle.
User Experience (UX) Design: UX design skills are essential to deliver a seamless and intuitive self-service experience. UX designers can create visually appealing and user-friendly interfaces, optimise navigation flows, and ensure consistency across different channels and touchpoints. UX designers focus on improving usability and addressing all variations of accessibility needs. More broadly, they need to lead the conversation of finding practical alternatives to those with limited/no digital access and those with low/no digital skills.
Conversational Design: Designing self-service conversations is an emerging skill that can now be officially accredited. It involves understanding user needs and preferences, creating conversational flows that are intuitive and user-friendly, and designing natural language interactions that align with user expectations. Conversational designers should deeply understand user psychology and be skilled in crafting effective dialogue and user journeys.
Data Analytics and Insights: This is focused on understanding user behaviour and pain points, tracking existing use cases, and identifying new ones. Sources include tracking metrics, mining logs from conversational AI solutions, and user research. Their actionable insight drives the overall team agenda.
Customer Journey Mapping: Effective self-service starts with understanding the customer journey, including pain points and moments of truth. This requires skills in journey mapping, data analysis, and empathy to understand the customer's perspective. The key goal is to reduce complexity so that a journey becomes simple enough to hand over to customers through redesign.
Machine Learning and AI: As self-service platforms increasingly use AI and machine learning for chatbots, recommendation engines, and automated processes, it's essential to understand these technologies. This includes technical development skills and the strategic understanding of where and when to implement them.
Natural Language Processing (NLP): NLP enables accurate understanding and interpretation of user inputs. NLP specialists can help train AI models for specific customer engagement use cases. This has been important in conversational AI solutions and will continue as generative AI becomes fused into self-service solutions.
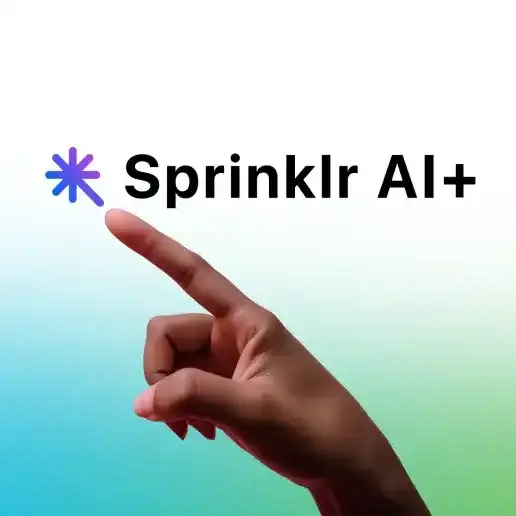
Knowledge Management: Building and maintaining a robust knowledge management system is crucial for self-service success. This includes knowledge curation, content organisation, and continuous updating of information. AI-based solutions are becoming more deeply involved in these tasks, allowing people to move into more of a supervisory role.
Continuous Improvement and Iteration: Self-service needs constant development. There is maintenance and optimisation of existing use cases. Then, discovery, design, testing and launching of new ones. Skills in agile methodologies, user testing, and rapid prototyping help iterate and refine self-service solutions.
Change Management and User Adoption: Successful implementation of self-service requires effective change management skills. This includes driving user adoption, managing user expectations, and providing training and support for both customers and colleagues.
Concluding Thoughts
Self-service ought to be the dominant source of customer service resolution. Currently, this is only true in top-performing organisations. Their success is less about their choice of technologies than their overall approach. Much of which I’ve covered in this exploration.
This is a foundation that needs to be built in every organisation. Blended human and virtual engagement will only grow in scope and sophistication, as hinted by the extraordinary talents already on show from Generative AI, avatars, and predictive intelligence. It’s time to get the basics in place.
