The next generation of CCaaS is here
Digital-first customer service, enterprise-scale voice support. Redefine customer service with an AI-powered platform that unifies voice, digital and social channels. Power channel-less interactions and seamless resolution no matter the channel of contact.
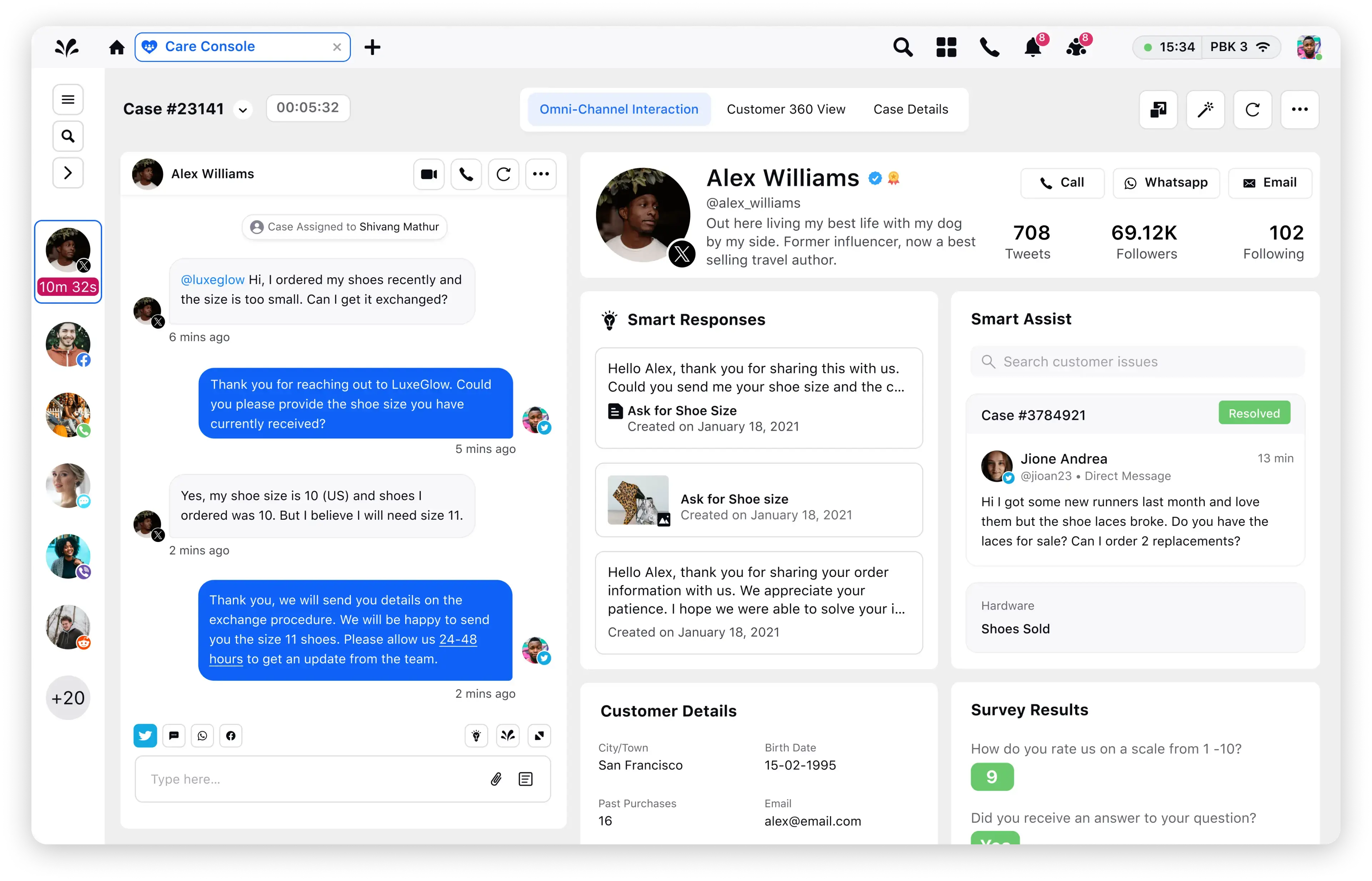
NLU engine benchmarking: a data-driven approach for AI market leaders
Natural Language Understanding (NLU) engines are massive customer sentiment drivers. AI and NLU evolved so much that a Google employee grabbed global attention when he claimed the company’s chatbot LaMDA was a self-aware human.
But don’t worry. We’re not here to spook you with stories of AI bots taking over the world, or customer service.
About 71% of American consumers still prefer a human touch in their customer service conversations, and that’s where benchmark NLU engines enter the picture.
NLU can help agents understand and serve customers better by adding layers of knowledge, context, and sentiment to customer interactions. Powered by benchmark NLU engines, conversational AI allows brands to be more intelligent and empathetic and spot hidden customer cues to make customer service more personal and less machine-like.
But how do you benchmark NLU engines to evaluate their AI capabilities? To get there, let’s first understand key technical terms.
- NLU engine benchmarking glossary
- NLU engine benchmarking: understand the process
- NLU engine benchmark report: the result
- Sprinklr emerges as a clear winner in NLU engine benchmarking
- Limitations of the NLU engine benchmarking
- Qualities that characterize top-performing NLU engines
- What makes Sprinklr’s NLU engine a market leader in conversational AI?
NLU engine benchmarking glossary
- Conversational AI
Conversational AI is an NLU-powered capability that enables computers and digital applications to engage customers with empathy by recognizing emotion, urgency, and context underlying human conversations. - Data set
A data set is a collection of related sets of information that computers can process as a single set of information. - Utterance
Utterance is a phrase or sentence of user speech received through text, audio, or video. NLU engines use utterances to train, test, and interpret user intents. - Intent
Intent indicates a user’s objective behind actions, events, or statements. For instance, a user action can be categorized as a product inquiry, complaint, refund request, etc. - Accuracy
Accuracy is the percentage of test sentences matched with the right intent by the NLU engine. - F1 Macro
The harmonic mean of the macro averages of precision and recall for each intent is called F1 Macro.
Precision = number of true positive results towards an intent divided by (/) all positive results towards an intent.
Recall = number of true positive results towards an intent divided by (/) number of results identified as positive towards an intent
NLU engine benchmarking: understand the process
Comparing NLU engines can be a tedious process. It can be time-consuming to shortlist a set of NLU-enabled solutions and go through the drill of testing the common intents observed in your customers. That’s where a structured approach backed by research comes in handy to evaluate NLU engines and their AI intuition capability with a bias-free approach.
Benchmarking natural language understanding services for building conversational agents
This NLU benchmarking method compares NLU engines on the dataset for a home automation bot broken down into small and large data sets to evaluate machine learning accuracy over different training and testing data sizes.
Methodology used in the NLU benchmarking method
Small data set
- 64 different intents are randomly picked
- 10 example sentences are used for each intent to train the NLU engine
- 1,076 example sentences (that are not a part of the training set) are tested
Large data set
- The same 64 intents mentioned above are picked for the large data set
- About 30 example sentences are used for each intent to train the NLU engine
- 5,518 example sentences (that are not part of the training set) are tested
NLU engine benchmark report: the result
The NLU benchmarking method shows Sprinklr’s NLP accuracy by virtue of recall and F1 macros to be well above its contemporaries — Google Cloud, Azure Language Studio, and AWS Comprehend. The benchmarking data and results can be found here.
If we break the NLU engine benchmarking down to small and large data sets, the Sprinklr NLU engine is still a clear winner.
Note: Larger data sets are the best way to test and train intents for higher accuracy. But the variation in accuracy with Sprinklr’s NLU engine is only ≤ 3%.
Small data set
Parameters:
- 640 training sentences - 10 sentences per Intent
- 1,076 test sentences
Large data set
Parameters:
- 1908 Training Sentences -~ 30 Sentences per Intent
- 5,518 test sentences
Sprinklr emerges as a clear winner in NLU engine benchmarking
Sprinklr’s NLU engine stays consistent and accurate in determining the intent of queries, with better mapping between test inputs and training inputs.
Example 1: Small data set
Query: is there anything i need to be aware of
Ground truth: calendar_query
Example 2: Large data set
Query: how many countries are in the European Union
Ground truth: qa_factoid
Limitations of the NLU engine benchmarking
- Size of the data set: Since a large number of well-researched data sets was used, NLU engines may have learned from the test utterances more quickly than was the case with raw, structured data found typically.
- Languages used: Only English was used to test different instances and intents.
- Nature of test data: The user utterances may not sound like typical customers, who could make more grammatical errors and have conversation gaps.
Qualities that characterize top-performing NLU engines
The cognitive abilities of NLU engines are just one of the factors to consider while evaluating them for your company. It helps overcome the tedious manual effort that stands in the way of understanding user intent at scale.
In addition, here are some more important qualities to look out for in an NLU engine:
1. Speed
The NLU engine has to turn in results quickly, as conversational AI is about understanding customer intent to respond with speed and accuracy. The speed of processing a customer interaction shouldn’t decrease the intent-detection accuracy of the NLU engine.
2. Verticalization
NLU engines have a multitude of use cases spanning industries such as technology, retail, e-commerce, logistics, and hospitality. The conversational AI functionality should be able to distinguish between these industries and adapt to every solution area with a unique approach.
3. Ease of use
Look out for NLU engines that are inclusive of non-technical employee profiles. Understanding how to test and train data sets shouldn’t be limited to quality assurance engineers and developers. It’s something business owners with a non-tech background can do by themselves. Conversational AI powered by no-code NLU engines is the way to improve adoption and usability.
4. Scalability
With more and more data inputs that an NLU engine gathers, it has to train itself in various regional semantics, linguistic variations, and different entities of user expression. Build an NLU framework that can process multiple languages and future-proof your conversational AI chatbots.
What makes Sprinklr’s NLU engine a market leader in conversational AI?
Sprinklr’s AI engine is purpose-built to understand and contextualize the entire spectrum of customer experience management. Here are seven differentiators that set Sprinklr AI apart from conventional conversational AI platforms:
1. Accurate message classification
Automatically read, decipher, and analyze customer messages, classify them as intents, and define internal teams for accurate case assignment.
2. Diligent crisis detection
Trigger alerts when customer interactions get out of hand using predetermined parameters such as negative brand mentions and keywords or AI-identified signs of distress such as sentiment detection.
3. Context-aware virtual assistance
Generate automated responses to customers or provide AI assistance to agents based on available customer data, knowledge base, and history of interactions across channels.
4. Future-ready predictive analysis
Foresee not just customer service but also market trends such as popular topics, macroeconomics, consumer sentiment, PR crises, and changing industry benchmarks to realign your product and marketing roadmaps. Sprinklr’s AI can recognize patterns across digital channels, customer demographics, and more with contextual data breakdowns.
5. Smart visual interpretations
Process visual data involved in brand and customer interactions to define images and videos accurately without a human agent.
6. End-to-end AI studio
Train, test, and deploy AI models within Sprinklr for better social listening, message classification, conversational AI and chatbots, response automation, and self-serve communities.
7. Brand interaction moderation
Monitor every agent-customer interaction to ensure adherence to internal brand guidelines and generate reports to identify areas of improvement for increasing customer satisfaction (CSAT) and reducing top contact drivers.
Do you want to scale your customer support with zero-touch personalization and operational efficiency? Sprinklr’s NLU engine can be the bridge you need — it comes with millions of AI predictions, data points, and hundreds of instantly deployable AI models.
