The next generation of CCaaS is here
Digital-first customer service, enterprise-scale voice support. Redefine customer service with an AI-powered platform that unifies voice, digital and social channels. Power channel-less interactions and seamless resolution no matter the channel of contact.
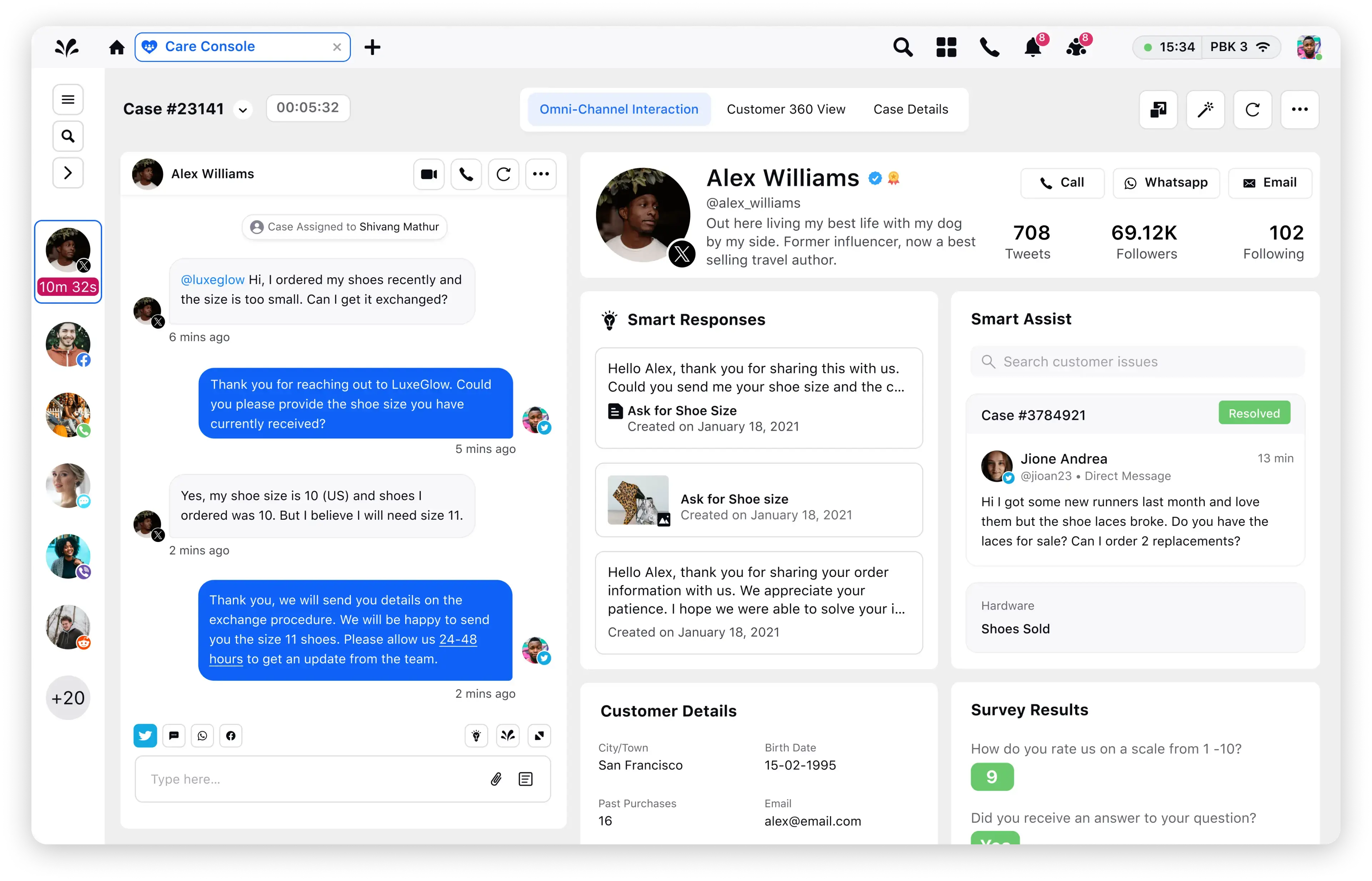
Launching Your Customer Service Chatbot: Strategies for the AI Era
In the age of instant messaging, who has the time — or patience — to dial a contact center and sit through hold music just to reset a password or check order status? For most customers today, that’s more frustrating than the problem they’re trying to solve. And agents often bear the brunt of that frustration as they are the first line of support.
To meet rising customer expectations — and protect brand loyalty — enterprises needed a solution that could handle these everyday interactions with speed, consistency and reliability. That’s where the customer service chatbot first came in: a digital tool designed to simulate human-like conversations and resolve routine service issues without human intervention.
However, the role of the chatbot has rapidly evolved. According to Gartner, by this year, 80% of companies are already using — or planning to use — chatbots in their customer service strategy. And with generative AI now at the forefront, these bots are far from basic. They’re smarter, more conversational and capable of handling complex queries beyond their original scope.
In this blog, we’ll unpack everything you need to know about customer service chatbots — from their types and capabilities to implementation best practices and the strategic advantages they bring to digital-first customer service.
What is a customer service chatbot?
A customer service chatbot is an intelligent virtual assistant built to simulate human-like conversations and provide automated support through text, voice, or both. These bots are designed to handle a wide range of service interactions, from resolving routine queries like order status or password resets to offering personalized recommendations based on customer behavior.
But not all chatbots are created equal.
Earlier generations of chatbots were rule-based, meaning they followed predefined scripts and decision trees. They could only respond to specific keywords or phrases — and once a customer stepped outside that script, the experience quickly fell apart.
Modern AI-powered chatbots are a different breed. Built on natural language processing (NLP) and machine learning, these bots can interpret intent, understand context and learn from every conversation. As a result, conversations feel more human, helpful and flexible — especially as they evolve with customer behavior and feedback. Take a look at the core differences between the two.
Rule-based chatbot | AI chatbot |
Follow predetermined decision trees with scripted responses | Learn and improve from each interaction |
Limited to answering questions within their programmed scope | Can handle nuanced, conversational interactions |
Require manual updates to expand capabilities | Capable of managing multiple, changing topics within a single conversation |
Easier to develop but less flexible in application | Provide personalized responses based on user data and context |
Unsurprisingly, 82% of consumers opt for chatbots when they want immediate answers, signaling a broader shift toward AI-first customer service. For global enterprises, the value is clear: round-the-clock support that’s scalable, multilingual and consistent — no matter the time zone.
How large enterprises benefit from customer care chatbots
For enterprise customer service leaders, delivering fast, scalable and personalized service is no longer a competitive edge today — it has now become a customer expectation. And AI-powered customer service chatbots are becoming essential to meet that demand.
1. Cost reduction at scale
One of the most immediate advantages of deploying customer service chatbots is cost savings. Chatbots automating high-volume, low-complexity interactions free up live agents for more complex and high-value conversations.
According to Gartner, conversational AI deployments are expected to cut agent labor costs by $80 billion by 2026. Chatbots can manage thousands of interactions simultaneously without fatigue, allowing enterprises to scale support operations without proportional increases in headcount or overhead.
2. Faster response times across channels
Customers today expect real-time resolutions — regardless of time zones or business hours. AI chatbots deliver instant, 24/7 responses, meeting customers wherever and whenever they reach out. By 2027, chatbots will be the primary customer service channel for 25% of organizations, underscoring a shift toward AI-led customer engagement models.
3. Enhanced customer experience
Customer service chatbots can deliver customized interactions based on customer data and previous engagements. This level of personalization helps you enhance customer satisfaction and build customer loyalty.
4. Deeper customer insights from real conversations
Every chatbot interaction is a data opportunity. Chatbots help customer service teams uncover patterns and proactively address friction points by capturing customer sentiments, preferences and recurring issues. These insights can inform product improvements, advertising campaigns and CX strategy — turning every conversation into a feedback loop for innovation.
📚 Read More: How Leading Brands are Using Messenger Bots
Key features to look for in customer service chatbots
When evaluating a modern customer service chatbot, the key question isn’t just “Can it respond to customers?”— but “Can it scale, adapt and deliver value across the entire service journey?”
Here are the must-have features that define enterprise-grade AI chatbots:
- Natural language understanding (NLU) and multilingual support
Modern chatbots go beyond keyword detection — they understand customer intent, emotion and context. NLU, powered by machine learning and NLP, enables bots to handle complex queries with nuance. Enterprise-grade chatbots also support multilingual conversations, ensuring seamless support across regions and demographics.
- Omnichannel deployment
Today’s customers don’t stick to one channel, nor should your customer service chatbot. Whether it’s your website, mobile app, WhatsApp, Instagram, SMS, or live chat widget, modern customer service chatbots deliver a unified experience across channels, maintaining context and conversation history throughout.
- Real-time escalation to human agents
Smart bots know their limits. When a conversation needs a human touch — like billing disputes, escalations, or emotionally sensitive topics — the chatbot should seamlessly hand off the case to a live agent with full context.
- Personalization and contextual memory
The most effective chatbots create the impression that they understand your customers. They access relevant information from various sources, including contact center CRM systems, customer data platforms, knowledge bases, chat history, and purchase records to personalize real-time conversations. Some advanced chatbots can also maintain context across different sessions and seamlessly send messages via SMS, email and WhatsApp during interactions, providing a continuous and connected experience.
- AI Training and feedback loops
Modern bots get smarter over time. With built-in machine learning and performance analytics, they constantly evolve based on customer feedback, escalation rates and success outcomes —turning every conversation into a learning opportunity.
Key performance metrics to track
To ensure your customer service chatbot is doing its job, track these high-impact metrics:
Metric | Why it matters |
Containment rate (or self-service rate) | Measures how many customer queries are fully resolved by the bot without human intervention — a direct indicator of chatbot efficiency. |
Average response time | Instantaneous replies drive better CX. This metric benchmarks how fast your bot responds across channels. |
Escalation rate | Tells you when and why customers are being handed off to agents — key to identifying training gaps or edge cases. |
Many enterprises now embed CSAT or thumbs-up/thumbs-down ratings directly after bot interactions to track real-time customer sentiment. | |
Intent recognition accuracy | Gauges how well your bot understands what customers are actually asking. If you witness low accuracy, refining training data can help. |
Cost per resolution | A powerful way to tie automation back to financial impact. Compare this with live agent costs to showcase ROI. |
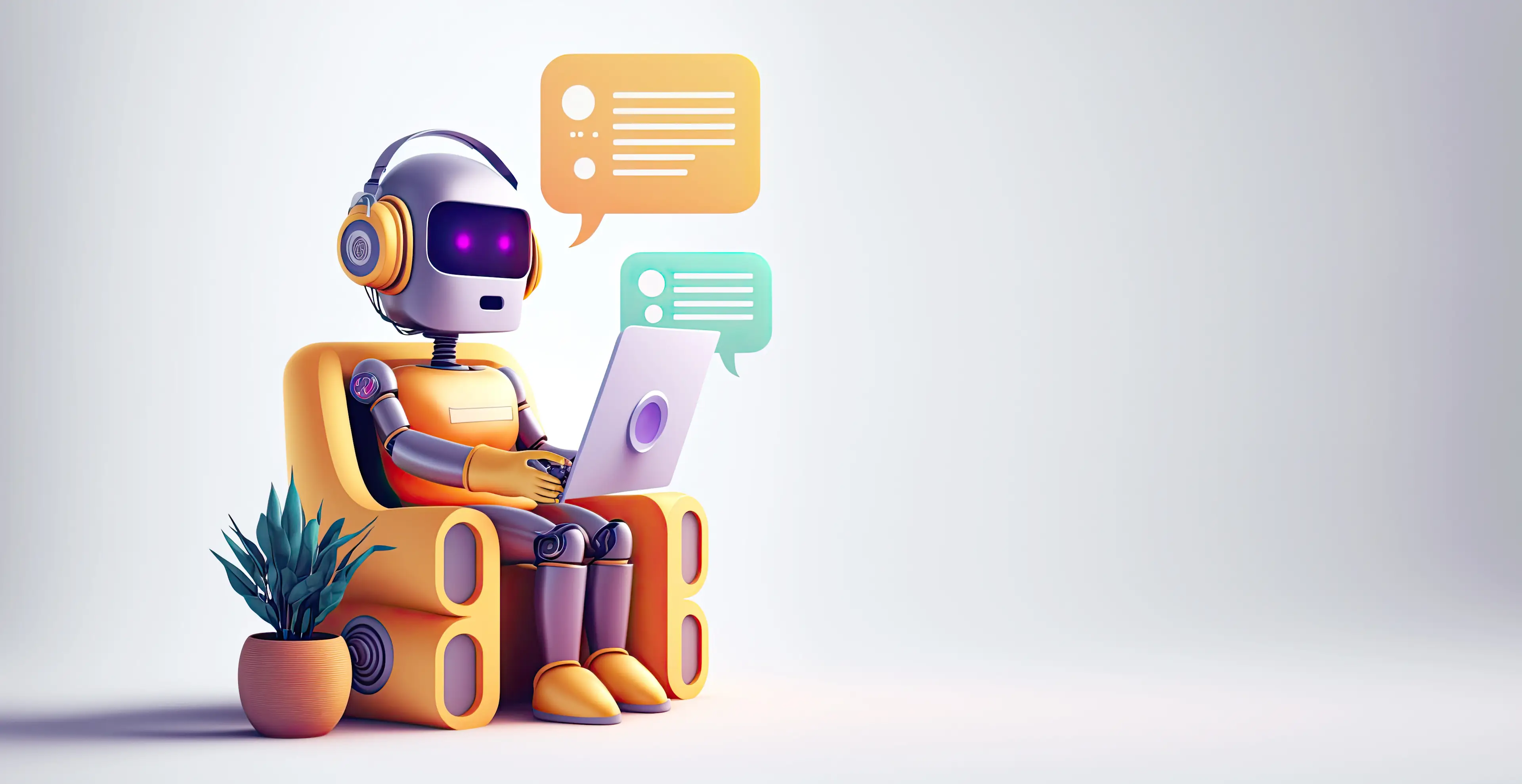
How to implement and optimize customer service chatbots (+ Best practices)
Rolling out a customer service chatbot isn’t just about plugging in a tool. At the enterprise level, it’s about reimagining how your service function operates — with scale, speed and intelligence at the core.
1. Start with the "Why"— Not the widget
Don’t start with, “We need a chatbot.” Instead, begin with, “What problem are we trying to solve?”
Is your contact center overwhelmed by repetitive queries? Are your service levels lagging outside business hours? Are agents burning out on low-value work?
Answering these questions upfront sets the right foundation. Without this clarity, you risk building a chatbot that’s either too generic or completely misaligned with customer pain points.
2. Design the chatbot around actual customer behavior
You don’t need to guess what customers want from your bot. You already have the data — buried in helpdesk tickets, CRM logs, live chat transcripts and IVR records. Extract that. Analyze it. Look for the 20–30 highest-frequency intents and design your chatbot to cover those flows from day one.
This intent-led approach makes your chatbot valuable on day one and leaves room for continuous training and refinement.
3. Involve your frontline team in design conversations
Your agents are on the frontlines for a reason — they know what customers ask, where the friction lives, and how to respond with empathy and nuance. If you’re building a chatbot in a vacuum, you’re missing a goldmine of context.
Loop in your frontline team early. Use their expertise to shape the conversational tone, flag policy or process gaps and stress-test logic flows. They’ll help you craft responses that sound more human, feel on-brand and guide customers to resolution — not confusion. Plus, their involvement builds trust internally. Your agents see the chatbot not as a threat but as a strategic support tool that reduces repetitive workload.
💡Do you know
With Sprinklr’s Conversational AI platform, building a chatbot is a no-code, 3-step process powered by simple natural language prompts. And to take it further, Sprinklr’s Generative-AI-powered dialog builder enables you to design, update, and scale conversation flows in minutes — without a single line of code. It’s intuitive enough for your frontline team to co-create and robust enough for enterprise-scale deployment.
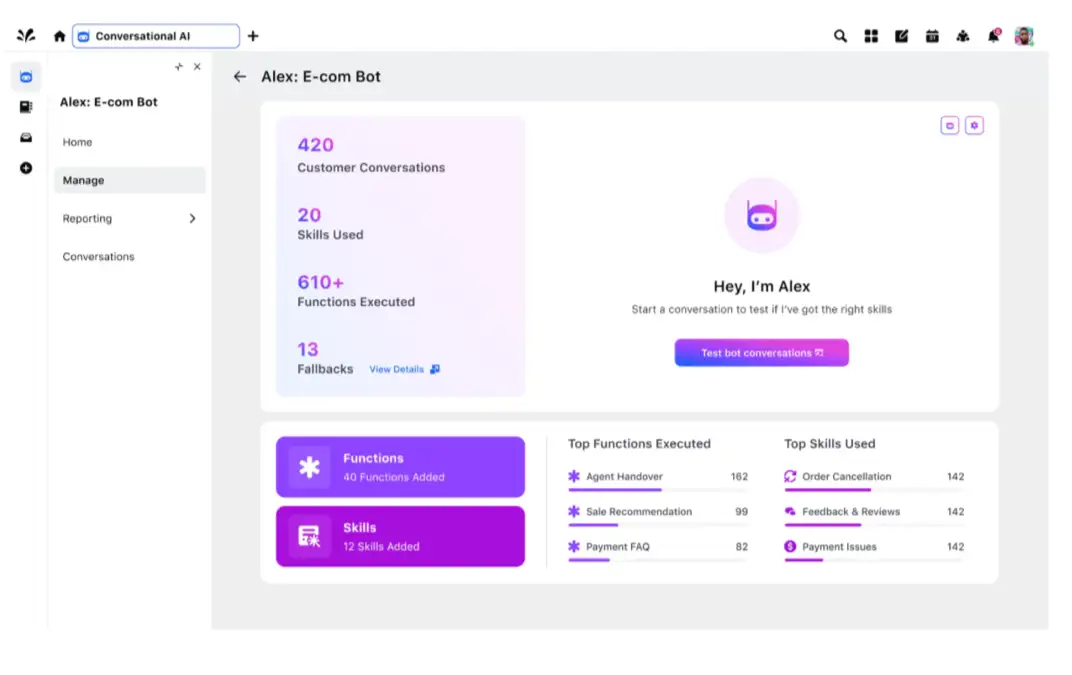
4. Build on a tech stack that supports real-time integration
To truly resolve issues, your chatbot needs more than scripts and NLP. It needs access to live backend systems like order management, billing, CRM, appointment scheduling and knowledge bases.
Ensure your chatbot platform supports APIs, real-time lookups and secure data handling. This is the difference between a “sorry, I can’t help with that” bot and one that can truly take action on the customer’s behalf.
5. Rigorously test your bot before going live
A chatbot’s value hinges on how well it performs when customers actually interact with it. And in enterprise scenarios, there’s little room for error. Before launch, run simulations using real customer queries — not just scripted test cases. Check for logic breakages, tone inconsistencies, edge case failures and how the bot handles escalation scenarios.
Test across all platforms where the bot will be deployed — website, app, messaging channels — and ensure consistent behavior and experience across the board. If your bot integrates with backend systems like CRM or order management tools, verify how it behaves when these systems are slow or fail.
6. Seamless escalation is non-negotiable
Let’s be clear: not every customer issue can or should be handled by a bot. But the handoff to a live agent needs to be frictionless.
When escalation happens, your chatbot must move forward with the context. The last thing customers want is to repeat themselves. This means integrating the bot into your agent desktop, ensuring transcripts, query history and customer data are transferred in real-time.
Think of the bot and the agent as co-owners of the same conversation — not two disconnected endpoints.
7. Establish continuous learning loops
Customer expectations change. So should your chatbot. A successful implementation includes feedback mechanisms, performance monitoring and a clear process for identifying broken flows or high-failure intents.
Set up a monitoring team — ideally a cross-functional squad of CX leads, analysts and bot trainers — to review metrics like:
- Containment rate (how often the bot resolves without escalation)
- Customer satisfaction with bot-only resolutions
- Intent recognition accuracy
- Fall-back rates and common failure points
Use this data to refine the bot’s logic and language continuously.
8. Don’t ignore governance, compliance and tone
Chatbot compliance can't be an afterthought for large enterprises — especially in regulated industries. Data retention, GDPR, CCPA, PCI-DSS — your chatbot must operate within your organization's regulatory framework.
Just as important is tone. Your bot’s voice should sound like your brand. Warm and conversational? Direct and efficient? Whatever it is, it must be consistent — and built into every interaction.
📌 Thank Us Later: 5 Ways to Determine the Right Customer Service Tone and Voice
Best practices for customer service chatbot deployment in the AI era
Best practice | Why it matters |
Start with high-volume, low-complexity queries | These are your chatbot’s low-hanging fruit — think of order status, password resets, or FAQs. They’re easy to automate, generate quick ROI and give your team confidence before scaling up. |
Train with enterprise-specific language and data | General LLMs are powerful, but they don’t speak your brand’s language out of the box. Feed them your support transcripts, product catalogs, tone guidelines and regulatory FAQs to make responses relevant and safe. |
Layer chatbot escalation with seamless agent handoff | Bots shouldn’t be black holes. When intent or sentiment flags complexity, the transition to a live agent should feel natural — with full context passed along. This is key to protecting CX and minimizing customer frustration. |
Monitor conversation quality with human-in-the-loop (HITL) mechanisms | AI learns from feedback. Use real-time QA dashboards, supervisor alerts and analyst reviews to refine bot behavior and prevent hallucinations. This is mission-critical in regulated or high-stakes industries. |
Establish a governance framework | AI chatbots aren’t set-it-and-forget-it. Define ownership across IT, customer service, legal and compliance teams. Set review cadences for training data, escalation thresholds and performance KPIs. |
Measure more than just deflection | Yes, cost savings are important. But also track CSAT for chatbot sessions, containment rate, escalation reasons and accuracy. Use these metrics to tell a full story of business impact. |
Continuously improve with real user data | Launch is just the beginning. Leverage interaction data to iterate on intents, refine response quality and discover new automation opportunities. Generative AI models thrive on a feedback loop. |
Bookmark Now: Enterprise Chatbots: The Ultimate Guide for 2025
The future of customer service chatbots (in 2025 and beyond)
Going forward, chatbots will become intelligent service agents — capable of not just solving queries but anticipating them. With tighter integration into enterprise data stacks, bots will proactively reach out to customers when they detect anomalies (like delivery delays or failed payments) or even remind them of renewals and product usage tips before they ask.
Here’s what’s shaping the next frontier of chatbot innovation:
- Gen AI-powered contextual intelligence
Customer service bots are evolving from intent classifiers to full-scale dialog agents. They’ll understand sentiment, emotion, historical context and even multi-turn conversations better than ever before — mimicking human intuition at scale.
- AI agents with embedded actionability
We’re entering the era of agentic AI. That means chatbots won’t just recommend — they’ll act. Whether it's modifying a subscription, initiating refunds, or updating CRM records, bots will have the secure backend access needed to resolve issues end-to-end.
- Multimodal and voice-native experiences
The future is not just text. Think video-based support, voice bots integrated into smart devices, or even avatar-based digital agents offering concierge-like assistance — all powered by AI models that understand tone, intent and visual cues.
- Bots as internal service enablers
Beyond customer-facing use cases, enterprises are deploying chatbots internally — to assist agents, pull up knowledge base articles in real-time, or even automate coaching. Learn more about agent assist software. This dual-use evolution drives productivity across the service value chain.
- Stronger governance, ethical AI and explainability
With great power comes great responsibility. Enterprises will prioritize transparency, bias mitigation and compliance frameworks as Gen AI matures. Chatbot decisions will need to be traceable — especially in industries like healthcare, finance and government services.
Build your customer service chatbot with best-in-class AI
Most enterprises don’t have a chatbot problem — they have a capability gap.
Legacy bots were built to deflect, not to serve. Rule-based scripts masquerading as “AI” often fall short in real conversations, leaving customers frustrated, agents overwhelmed and CX leaders caught in the middle.
Today’s enterprise needs intelligent automation that earns trust, scales naturally, and delivers resolution — not redirection.
That’s where Sprinklr steps in.
Sprinklr AI powered by proprietary large language models (LLMs), 750+ out-of-the-box intent models and pre-trained industry datasets is designed for real enterprise use cases.
With Sprinklr's Conversational AI, you can:
- Automate 70%+ of inbound conversations with human-like empathy and precision
- Deploy multilingual bots across voice, chat and messaging channels, all from one unified workspace
- Ensure smart handoffs to agents when needed — complete with full context
- Enable bots to take real action — from booking appointments to processing refunds — not just answer FAQs
- Gain real-time insights into customer intent, sentiment and emerging issues with native AI-powered analytics
And with Sprinklr AI+, you’re not just getting Gen AI layered onto existing systems — you’re unlocking a proprietary AI engine purpose-built for customer experience, with enterprise-grade governance, transparency and security baked in.
In the AI era, every second counts, and every interaction matters. Don’t settle for a chatbot that talks. Build one that understands. Take the first step by booking an expert-led demo.
Frequently Asked Questions
To integrate AI-powered chatbots, you'll want to leverage APIs and SDKs to establish seamless connections with your current CRM, help desk software and messaging platforms. It's crucial to ensure data synchronization across all systems to maintain a unified view of each customer.
The success of customer service chatbots can be evaluated by tracking metrics such as resolution rate, customer satisfaction (CSAT) scores and the average time to resolution. Also, pay attention to the frequency with which chatbots escalate queries to human agents
Yes, customer service bots enhance personalization by utilizing customer data and preferences to tailor interactions. They facilitate real-time engagement by providing instant responses and 24/7 availability.
AI customer service bots analyze customer interactions and identify trends using machine learning, which allows them to predict common issues and proactively offer solutions. These chatbots adapt to evolving customer needs by continuously learning from new data and feedback, thereby improving their accuracy and relevance over time.
Advanced customer service chatbots are equipped with natural language processing and knowledge bases, enabling them to handle a wide range of complex queries. They are also programmed to recognize when a query requires human intervention and can seamlessly escalate the conversation to a human agent, ensuring that the context of the interaction is preserved.
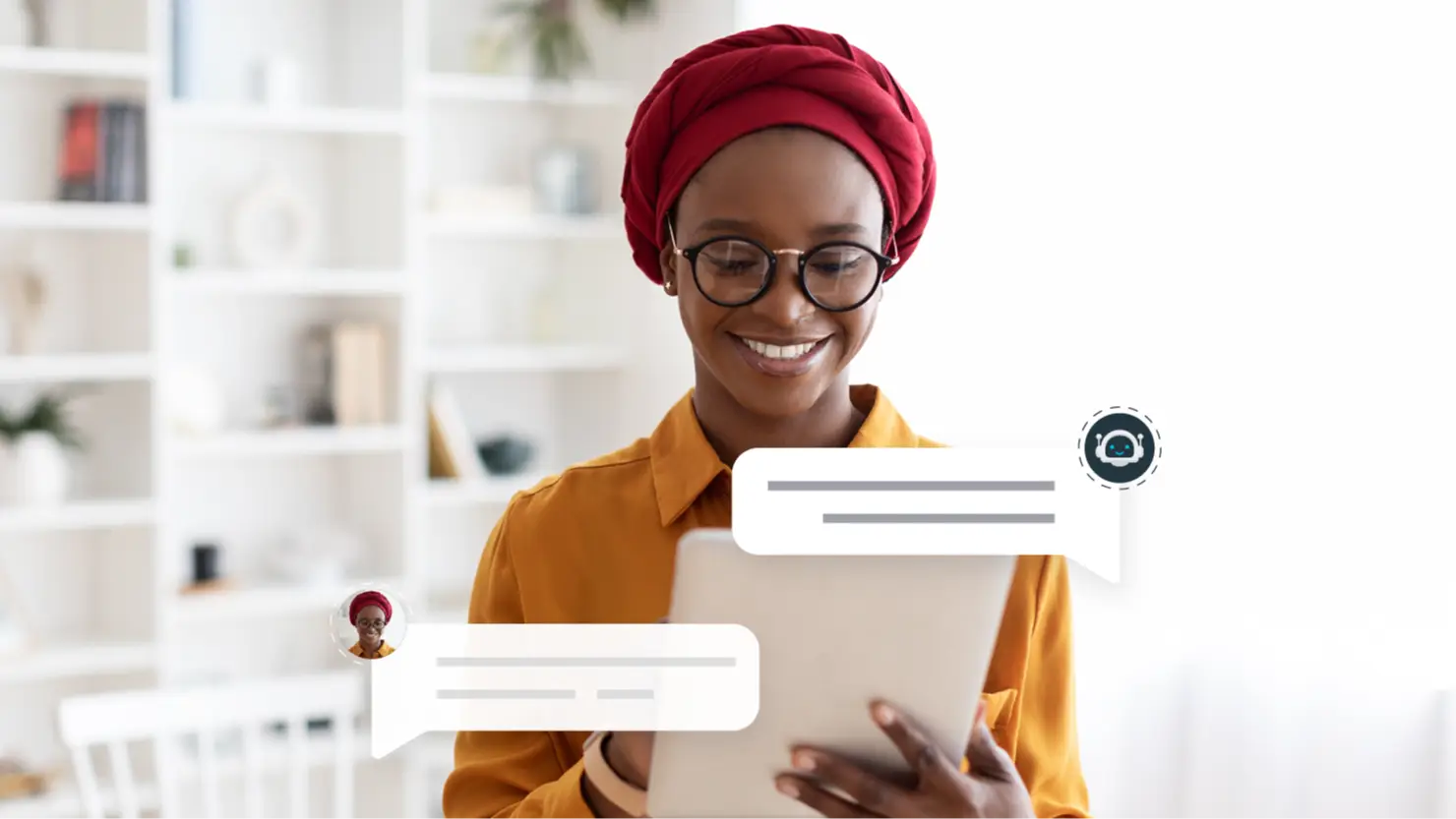